Why is transparency of data more important in data science than analysis?
Why is transparency of data more important in data science than analysis?
Do you want to be a data scientist?
If you want to be a data scientist, you’ve got to know the importance of transparency. This is one of the most common mistakes that people in your field make. It’s not just about reading examples on how to do detailed analysis or how to use certain software, but it also includes learning about the consequences of transparency. If this sounds new, don’t worry- it will be part of your training if you enroll in one of our master’s programs for data science!
There are benefits and drawbacks to transparency in all sorts of ways- from storing information with encryption or without, doing analysis with visualization or without, reading research papers without comprehension or with more depth needed. Transparency is a core concept in data science, and it has a lot to do with the importance of data analysis. Here are some examples of how transparency can affect a data scientist’s work:
Why is Transparency Important?
Transparency refers to being able to tell what something is made out of- all the different parts, as well as being able to look at the whole. This isn’t just important in data science, but in other fields, including chemistry and physics. Transparency allows scientists to look at both the parts and their relationship to one another.
When you don’t have transparency, there is a lot of data science that can’t be done. If you want to build models and learn information without being able to see the whole picture, it’s not possible. You will have to look at certain parts of the model in isolation or end up with incomplete information. This means that you will be unable to do a wide array of data science tasks, depending on what information is either muddled or missing.
The Importance of Transparency in Data Analysis
Transparency is the most important part of the analysis because it keeps you from making crucial mistakes while collecting and working with data. By knowing what data is available, how it was collected, and what the different categories represent, you can avoid any mistakes that can have a negative effect on your analysis. Without transparency, you will end up with inaccurate information or none at all.
Data transparency also helps to improve your models by allowing you to easily implement new algorithms when needed. There isn’t a sense of worry about the structure of your data because you already have the gist of it! This allows for quick changes based on new insights that can lead to better models.
What Should You Do Instead?
With transparency in mind, there are some data science tasks that require it more than others. When it comes to data science, there are more things and strategies to predict the behavior or relationships of data points in a data set.
If you want to know more about Data Science and its technologies, you can refer to the Data Science training in Kochi. Never stop learning, there may be plenty of free resources available, but an interactive session makes learning more effective and refers to free resources. Here you can check out the Data Science courses in Kochi.
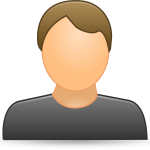